It’s been about two weeks since I came back from the adventure that is called the StartupBus. Things just start to sink in, soon the glare will fade and see what remains of the total awesomeness that is the Bus. I think a lot will stick with me, it was just way beyond what I could have imagined and I can already feel the changes the trip & the people made to me. The best possible changes, to say. :)
So, the StartupBus in a nutshell: 6 buses from all around the US set off a journey, with about 30 “buspreneurs” on board each, who ideally don’t know each other or haven’t worked together before. First, whoever has some business/product ideas, can pitch to the others. Groups are formed and everybody starts to make their idea into reality – and have to do that frantically, since there’s only about 48 hours before the buses arrive to their destination. Sounds like fun? If it doesn’t, you should just give it a try :)
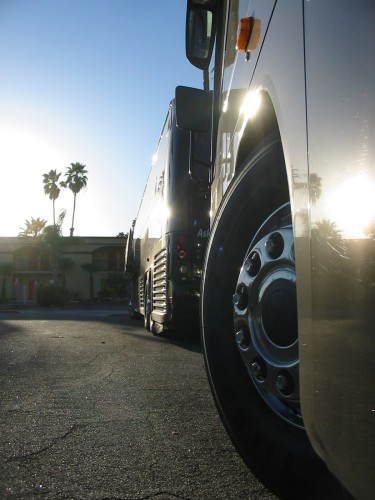
I had no idea what to expect and when I heard the pitches that Tuesday morning, rolling out of San Francisco on the highway, I was thinking to myself: what do I do here? What can I contribute? There are just so many amazing developers, setting the bar. But I could choose a project in the end, something that was close to my heart since I do love to travel: Fly By Miles (the site went back to pre-launch mode for a bit, but that’s where it will be ;) – a site that wants people to use their frequent flyer miles well and easily. Our team had 8 members altogether: 5 developers (with yours truly), 2 designers and a business strategist. Of course these are lines drawn in the sand, everyone chipped in a bit in each role.
I have to say, thos ~3 days are close to a blur now. Should have continued writing my journal but there was just no chance for that. We were mostly hopping from co-working spaces to hotels and back, all through California, Arizona, New Mexico and Texas. I should know where, I wrote the Android app that told the map where we all are. There were some very nice places, interesting scenery that I haven’t seen much of. There were challenges like walking up to people in Santa Monica and getting feedback on our project – and taking a video of it (before this I’ve never thought I could do that. But got our 3 recorded videos and a few people’s thoughts off-the-record). There was always something to do and I enjoyed being left to my own devices and coming back with a solution. Not the best solution usually, so there were usually more than one iterations for solving each of the problems I tried to tackle, but at least there was a good modus operandi: give Greg a task, some time, maybe a couple of Red Bulls and he’ll come back with something.
By the way, Red Bulls: don’t let them anywhere near me for the time being. Lost count at 7, but the shakes stayed for a couple days after I stopped so it must have been more than that. Haven’t had it since high school (which has been a while) and under this hackathon circumstances they are very addictive…
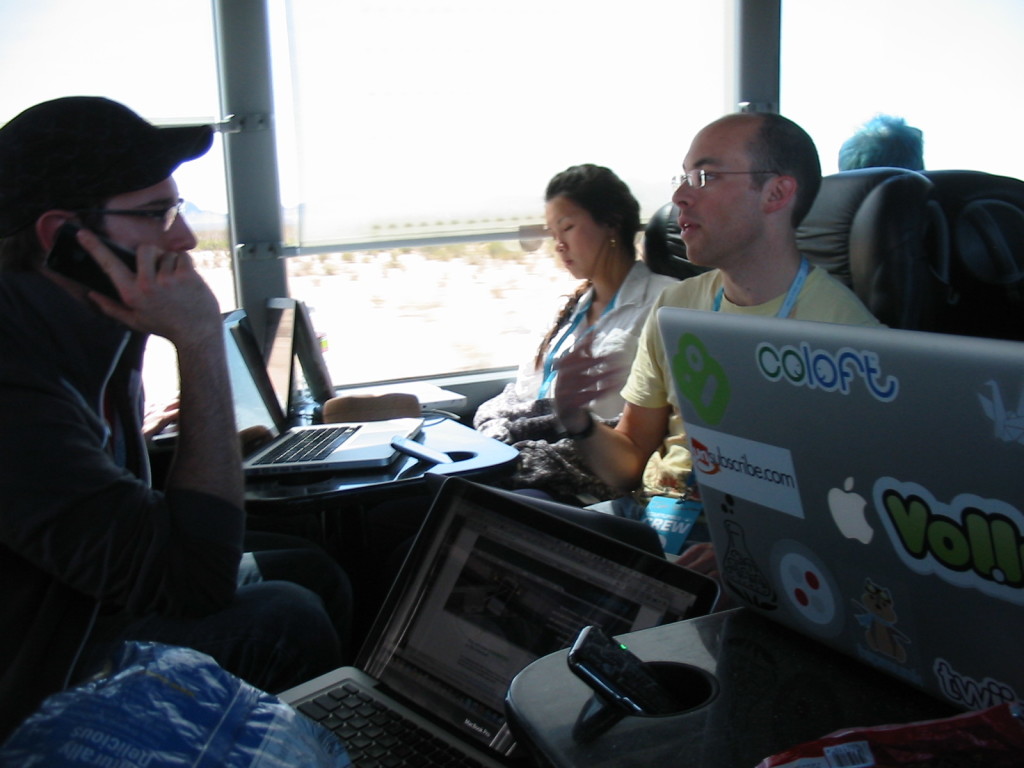
Well, after a few hotels and sleepless/frantic nights, we arrived to Austin. Finished up a proof-of-concept, working prototype and got some sleep. The next days were about taking Austin in, with Ignite, with SxSW, flashmob, friends, places, food… There was some programming as well, since Fly By Miles got into the semifinals, then into the finals, held in the Hilton. It was great, I really enjoyed it, an albeit we didn’t win, there was a lot to take home. Also, since that was my last night in Austin, I haven’t actually slept (again) and it was surreal to leave the place at 5am. Missed our CNN interview, but well, if we do it right, there will be more than enough to make up for that. ;)
What do I think I learned:
- Now I can code everywhere. In the park, on the curb, on the plane, on the bus… Though Taiwanese buses have more hectic drivers and their suspension is worse than the Bus was so better hold on to that computer. Haven’t been coding on a motor-scooter yet, but I will…. probably won’t try that. :)
- It is worth knowing the popular tech in the field I’m interested in even if I’m not using it (yet). In our group Ruby on Rails was the thing – and I’m a Python hacker. It was quite confusing for the first time, but I can see the advantages. This stands for other fields as well: mobile development, databases, design, front end interface…. There’s a lot of interesting tech out there, and I believe that one should try things out before the need arises. That makes educated choices down the road.
- Have a niche. I might be biased, but I think this is even more important than the previous point. Know something else than others. Networking, game development, Big Data, Python, functional programming, myriads of 3rd party APIs (Google, Twillio, …), and so on. Anything that excites you.
- Be quick learner. Before the trip I had to (well, wanted to :P ) pick up Android development in a week. And it produced something that crashed fewer times than I thought it would. :) After coming back I wanted to pick up Django in a week because I was curious. There’s even a result (Wanna see? There will be a proper post about that). I believe everyone can do that, so no excuse for not learning a new things ever week.
- Choose carefully in what things you rely on others. It is easy to get burned and few things are worse than having your fate in someone else’s hand.
- Take note what people say, but don’t have to take them too seriously. Everyone’s been excited at the end of the trip and wanted to continue the project. Two weeks passed and 2 (and a half) people left of the 8. No hard feelings, that’s how things work and there will certainly time to work with them together.
- Getting in touch with people is easy. Not just technically, but nothing should hold you back. Fire off that email to the big shot you met, talk to the 2nd level contact on Linked In if you need to… Most of them will love something personal, just keep it simple, honest, and no hard feelings if it doesn’t work out. This liberated me on so many levels
- Startup life is a test on one’s liver. I’d thought it is a marginal issue, but better be prepared. Not sure what way (no, I don’t mean training), but got to.
- The best is always what you have but it’s always good to look out for more. For example. the Silicon Valley bus was undoubtedly the best bus of them all! :D But that won’t stop me from knowing as many amazing folks from each of the buses and off the buses as well.
- Silicon Valley at least was Mac World. Been an outsider with my Lenovo, but I don’t mind if I cannot share the power plug with the other 29 peeps on the bus. Penguin power!
- Laptop stickers are cool. I’m late to the party to say that (seeing some of the laptops there) but anyways…. I got to be selective, though, otherwise just too many stickers flying around.
- Now I just cannot stop the flow of ideas. It is not a question that I’m a “starter”. What I need to learn how to select the good ones (or, probably I should select the “ridiculous” ones, they seem to work the best), and how to be a “finisher”.
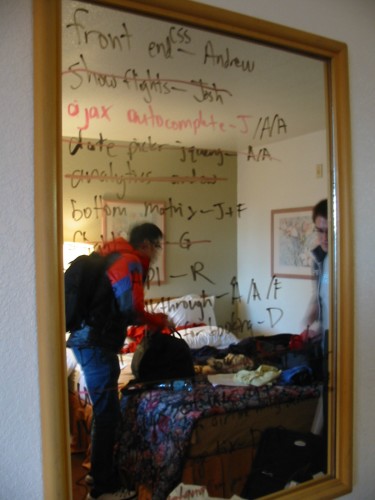
Probably there are more lessons, but that’s enough. Now off to do some practice. Let’s see what happens until next year’s Bus :)